A reminder for new readers. That Was The Week includes a collection of my selected readings on critical issues in tech, startups, and venture capital. I selected the articles because they are of interest to me. The selections often include things I entirely disagree with. But they express common opinions, or they provoke me to think. The articles are snippets sized to convey why they are of interest. Click on the headline, contents link, or the ‘More’ link at the bottom of each piece to go to the original. I express my point of view in the editorial and the weekly video below.
Hat Tip to this week’s creators: @leopoldasch, @JoeSlater87, @GaryMarcus, @ulonnaya, @alex, @ttunguz, @mmasnick, @dannyrimer, @imdavidpierce, @asafitch, @ylecun, @nxthompson, @kaifulee, @DaphneKoller, @AndrewYNg, @aidangomez, @Kyle_L_Wiggers, @waynema, @QianerLiu, @nicnewman, @nmasc_, @steph_palazzolo, @nofilmschool
Contents
Danny Rimer on 20VC - (Must See)
Leopold Aschenbrenner
Editorial
I had not heard of Leopold Aschenbrenner until yesterday. I was meeting with Faraj Aalaei (a SignalRank board member) and my colleague Rob Hodgkinson when they began to talk about “Situational Awareness,” his essay on the future of AGI, and its likely speed of emergence.
So I had to read it, and it is this week’s essay of the week. He starts his 165-page epic with:
Before long, the world will wake up. But right now, there are perhaps a few hundred people, most of them in San Francisco and the AI labs, that have situational awareness. Through whatever peculiar forces of fate, I have found myself amongst them.
So, Leopold is not humble. He finds himself “among” the few people with situational awareness.
As a person prone to bigging up myself, I am not one to prematurely judge somebody’s view of self. So, I read all 165 pages.
He makes one point. The growth of AI capability is accelerating. More is being done at a lower cost, and the trend will continue to be super-intelligence by 2027. At that point, billions of skilled bots will solve problems at a rate we cannot imagine. And they will work together, with little human input, to do so.
His case is developed using linear progression from current developments. According to Leopold, all you have to believe in is straight lines.
He also has a secondary narrative related to safety, particularly the safety of models and their weightings (how they achieve their results).
By safety, he does not mean the models will do bad things. He means that third parties, namely China, can steal the weightings and reproduce the results. He focuses on the poor security surrounding models as the problem. And he deems governments unaware of the dangers.
Although German-born, he argues in favor of the US-led effort to see AGI as a weapon to defeat China and threatens dire consequences if it does not. He sees the “free world” as in danger unless it stops others from gaining the sophistication he predicts in the time he predicts.
At that point, I felt I was reading a manifesto for World War Three.
But as I see it, the smartest people in the space have converged on a different perspective, a third way, one I will dub AGI Realism. The core tenets are simple:
Superintelligence is a matter of national security. We are rapidly building machines smarter than the smartest humans. This is not another cool Silicon Valley boom; this isn’t some random community of coders writing an innocent open source software package; this isn’t fun and games. Superintelligence is going to be wild; it will be the most powerful weapon mankind has ever built. And for any of us involved, it’ll be the most important thing we ever do.
America must lead. The torch of liberty will not survive Xi getting AGI first. (And, realistically, American leadership is the only path to safe AGI, too.) That means we can’t simply “pause”; it means we need to rapidly scale up US power production to build the AGI clusters in the US. But it also means amateur startup security delivering the nuclear secrets to the CCP won’t cut it anymore, and it means the core AGI infrastructure must be controlled by America, not some dictator in the Middle East. American AI labs must put the national interest first.
We need to not screw it up. Recognizing the power of superintelligence also means recognizing its peril. There are very real safety risks; very real risks this all goes awry—whether it be because mankind uses the destructive power brought forth for our mutual annihilation, or because, yes, the alien species we’re summoning is one we cannot yet fully control. These are manageable—but improvising won’t cut it. Navigating these perils will require good people bringing a level of seriousness to the table that has not yet been offered.
As the acceleration intensifies, I only expect the discourse to get more shrill. But my greatest hope is that there will be those who feel the weight of what is coming, and take it as a solemn call to duty.
I persisted in reading it, and I think you should, too—not for the war-mongering element but for the core acceleration thesis.
My two cents: Leopold underestimates AI's impact in the long run and overestimates it in the short term, but he is directionally correct.
Anthropic released v3.5 of Claude.ai today. It is far faster than the impressive 3.0 version (released a few months ago) and costs a fraction to train and run. it is also more capable. It accepts text and images and has a new feature that allows it to run code, edit documents, and preview designs called ‘Artifacts.’
Claude 3.5 Opus is probably not far away.
Situational Awareness projects trends like this into the near future, and his views are extrapolated from that perspective.
Contrast that paper with “ChatGPT is Bullshit,” a paper coming out of Glasgow University in the UK. The three authors contest the accusation that ChatGPT hallucinates or lies. They claim that because it is a probabilistic word finder, it spouts bullshit. It can be right, and it can be wrong, but it does not know the difference. It’s a bullshitter.
Hilariously, they define three types of BS:
Bullshit (general)
Any utterance produced where a speaker has indifference towards the truth of the utterance.
Hard bullshit
Bullshit produced with the intention to mislead the audience about the utterer’s agenda.
Soft bullshit
Bullshit produced without the intention to mislead the hearer regarding the utterer’s agenda.
They then conclude:
With this distinction in hand, we’re now in a position to consider a worry of the following sort: Is ChatGPT hard bullshitting, soft bullshitting, or neither? We will argue, first, that ChatGPT, and other LLMs, are clearly soft bullshitting. However, the question of whether these chatbots are hard bullshitting is a trickier one, and depends on a number of complex questions concerning whether ChatGPT can be ascribed intentions.
This is closer to Gary Marcus's point of view in his ‘AGI by 2027?’ response to Leopold. It is also below.
I think the reality is somewhere between Leopold and Marcus. AI is capable of surprising things, given that it is only a probabilistic word-finder. And its ability to do so is becoming cheaper and faster. The number of times it is useful easily outweighs, for me, the times it is not. Most importantly, AI agents will work together to improve each other and learn faster.
However, Gary Marcus is right that reasoning and other essential decision-making characteristics are not logically derived from an LLM approach to knowledge. So, without additional or perhaps different elements, there will be limits to where it can go. Gary probably underestimates what CAN be achieved with LLMs (indeed, who would have thought they could do what they already do). And Leopold probably overestimates the lack of a ceiling in what they will do and how fast that will happen.
It will be fascinating to watch. I, for one, have no idea what to expect except the unexpected. OpenAI Founder Illya Sutskever weighed in, too, with a new AI startup called Safe Superintelligence Inc. (SSI). The most important word here is superintelligence, the same word Leopold used. The next phase is focused on higher-than-human intelligence, which can be reproduced billions of times to create scaled Superintelligence.
The Expanding Universe of Generative Models piece below places smart people in the room to discuss these developments. Yann LeCun, Nicholas Thompson, Kai-Fu Lee, Daphne Koller, Andrew Ng, and Aidan Gomez are participants.
Essays of the Week
Situational Awareness: The Decade Ahead
Leopold Aschenbrenner, June 2024
You can see the future first in San Francisco.
Over the past year, the talk of the town has shifted from $10 billion compute clusters to $100 billion clusters to trillion-dollar clusters. Every six months another zero is added to the boardroom plans. Behind the scenes, there’s a fierce scramble to secure every power contract still available for the rest of the decade, every voltage transformer that can possibly be procured. American big business is gearing up to pour trillions of dollars into a long-unseen mobilization of American industrial might. By the end of the decade, American electricity production will have grown tens of percent; from the shale fields of Pennsylvania to the solar farms of Nevada, hundreds of millions of GPUs will hum.
The AGI race has begun. We are building machines that can think and reason. By 2025/26, these machines will outpace many college graduates. By the end of the decade, they will be smarter than you or I; we will have superintelligence, in the true sense of the word. Along the way, national security forces not seen in half a century will be unleashed, and before long, The Project will be on. If we’re lucky, we’ll be in an all-out race with the CCP; if we’re unlucky, an all-out war.
Everyone is now talking about AI, but few have the faintest glimmer of what is about to hit them. Nvidia analysts still think 2024 might be close to the peak. Mainstream pundits are stuck on the willful blindness of “it’s just predicting the next word”. They see only hype and business-as-usual; at most they entertain another internet-scale technological change.
Before long, the world will wake up. But right now, there are perhaps a few hundred people, most of them in San Francisco and the AI labs, that have situational awareness. Through whatever peculiar forces of fate, I have found myself amongst them. A few years ago, these people were derided as crazy—but they trusted the trendlines, which allowed them to correctly predict the AI advances of the past few years. Whether these people are also right about the next few years remains to be seen. But these are very smart people—the smartest people I have ever met—and they are the ones building this technology. Perhaps they will be an odd footnote in history, or perhaps they will go down in history like Szilard and Oppenheimer and Teller. If they are seeing the future even close to correctly, we are in for a wild ride.
Let me tell you what we see.
Table of Contents
Each essay is meant to stand on its own, though I’d strongly encourage reading the series as a whole. For a pdf version of the full essay series, click here.
Introduction
History is live in San Francisco.
I. From GPT-4 to AGI: Counting the OOMs
AGI by 2027 is strikingly plausible. GPT-2 to GPT-4 took us from ~preschooler to ~smart high-schooler abilities in 4 years. Tracing trendlines in compute (~0.5 orders of magnitude or OOMs/year), algorithmic efficiencies (~0.5 OOMs/year), and “unhobbling” gains (from chatbot to agent), we should expect another preschooler-to-high-schooler-sized qualitative jump by 2027.
II. From AGI to Superintelligence: the Intelligence Explosion
AI progress won’t stop at human-level. Hundreds of millions of AGIs could automate AI research, compressing a decade of algorithmic progress (5+ OOMs) into ≤1 year. We would rapidly go from human-level to vastly superhuman AI systems. The power—and the peril—of superintelligence would be dramatic.
III. The Challenges
IIIa. Racing to the Trillion-Dollar Cluster
The most extraordinary techno-capital acceleration has been set in motion. As AI revenue grows rapidly, many trillions of dollars will go into GPU, datacenter, and power buildout before the end of the decade. The industrial mobilization, including growing US electricity production by 10s of percent, will be intense.
IIIb. Lock Down the Labs: Security for AGI
The nation’s leading AI labs treat security as an afterthought. Currently, they’re basically handing the key secrets for AGI to the CCP on a silver platter. Securing the AGI secrets and weights against the state-actor threat will be an immense effort, and we’re not on track.
IIIc. Superalignment
Reliably controlling AI systems much smarter than we are is an unsolved technical problem. And while it is a solvable problem, things could easily go off the rails during a rapid intelligence explosion. Managing this will be extremely tense; failure could easily be catastrophic.
IIId. The Free World Must Prevail
Superintelligence will give a decisive economic and military advantage. China isn’t at all out of the game yet. In the race to AGI, the free world’s very survival will be at stake. Can we maintain our preeminence over the authoritarian powers? And will we manage to avoid self-destruction along the way?
IV. The Project
As the race to AGI intensifies, the national security state will get involved. The USG will wake from its slumber, and by 27/28 we’ll get some form of government AGI project. No startup can handle superintelligence. Somewhere in a SCIF, the endgame will be on.
V. Parting Thoughts
What if we’re right?
ChatGPT is bullshit
Original Paper, Open access, Published: 08 June 2024
Volume 26, article number 38, (2024)
Michael Townsen Hicks, James Humphries & Joe Slater
Abstract
Recently, there has been considerable interest in large language models: machine learning systems which produce human-like text and dialogue. Applications of these systems have been plagued by persistent inaccuracies in their output; these are often called “AI hallucinations”. We argue that these falsehoods, and the overall activity of large language models, is better understood as bullshit in the sense explored by Frankfurt (On Bullshit, Princeton, 2005): the models are in an important way indifferent to the truth of their outputs. We distinguish two ways in which the models can be said to be bullshitters, and argue that they clearly meet at least one of these definitions. We further argue that describing AI misrepresentations as bullshit is both a more useful and more accurate way of predicting and discussing the behaviour of these systems.
Introduction
Large language models (LLMs), programs which use reams of available text and probability calculations in order to create seemingly-human-produced writing, have become increasingly sophisticated and convincing over the last several years, to the point where some commentators suggest that we may now be approaching the creation of artificial general intelligence (see e.g. Knight, 2023 and Sarkar, 2023). Alongside worries about the rise of Skynet and the use of LLMs such as ChatGPT to replace work that could and should be done by humans, one line of inquiry concerns what exactly these programs are up to: in particular, there is a question about the nature and meaning of the text produced, and of its connection to truth. In this paper, we argue against the view that when ChatGPT and the like produce false claims they are lying or even hallucinating, and in favour of the position that the activity they are engaged in is bullshitting, in the Frankfurtian sense (Frankfurt, 2002, 2005). Because these programs cannot themselves be concerned with truth, and because they are designed to produce text that looks truth-apt without any actual concern for truth, it seems appropriate to call their outputs bullshit.
We think that this is worth paying attention to. Descriptions of new technology, including metaphorical ones, guide policymakers’ and the public’s understanding of new technology; they also inform applications of the new technology. They tell us what the technology is for and what it can be expected to do. Currently, false statements by ChatGPT and other large language models are described as “hallucinations”, which give policymakers and the public the idea that these systems are misrepresenting the world, and describing what they “see”. We argue that this is an inapt metaphor which will misinform the public, policymakers, and other interested parties.
The structure of the paper is as follows: in the first section, we outline how ChatGPT and similar LLMs operate. Next, we consider the view that when they make factual errors, they are lying or hallucinating: that is, deliberately uttering falsehoods, or blamelessly uttering them on the basis of misleading input information. We argue that neither of these ways of thinking are accurate, insofar as both lying and hallucinating require some concern with the truth of their statements, whereas LLMs are simply not designed to accurately represent the way the world is, but rather to give the impression that this is what they’re doing. This, we suggest, is very close to at least one way that Frankfurt talks about bullshit. We draw a distinction between two sorts of bullshit, which we call ‘hard’ and ‘soft’ bullshit, where the former requires an active attempt to deceive the reader or listener as to the nature of the enterprise, and the latter only requires a lack of concern for truth. We argue that at minimum, the outputs of LLMs like ChatGPT are soft bullshit: bullshit–that is, speech or text produced without concern for its truth–that is produced without any intent to mislead the audience about the utterer’s attitude towards truth. We also suggest, more controversially, that ChatGPT may indeed produce hard bullshit: if we view it as having intentions (for example, in virtue of how it is designed), then the fact that it is designed to give the impression of concern for truth qualifies it as attempting to mislead the audience about its aims, goals, or agenda. So, with the caveat that the particular kind of bullshit ChatGPT outputs is dependent on particular views of mind or meaning, we conclude that it is appropriate to talk about ChatGPT-generated text as bullshit, and flag up why it matters that – rather than thinking of its untrue claims as lies or hallucinations – we call bullshit on ChatGPT.
What is ChatGPT?
Large language models are becoming increasingly good at carrying on convincing conversations. The most prominent large language model is OpenAI’s ChatGPT, so it’s the one we will focus on; however, what we say carries over to other neural network-based AI chatbots, including Google’s Bard chatbot, AnthropicAI’s Claude (claude.ai), and Meta’s LLaMa. Despite being merely complicated bits of software, these models are surprisingly human-like when discussing a wide variety of topics. Test it yourself: anyone can go to the OpenAI web interface and ask for a ream of text; typically, it produces text which is indistinguishable from that of your average English speaker or writer. The variety, length, and similarity to human-generated text that GPT-4 is capable of has convinced many commentators to think that this chatbot has finally cracked it: that this is real (as opposed to merely nominal) artificial intelligence, one step closer to a human-like mind housed in a silicon brain.
However, large language models, and other AI models like ChatGPT, are doing considerably less than what human brains do, and it is not clear whether they do what they do in the same way we do. The most obvious difference between an LLM and a human mind involves the goals of the system. Humans have a variety of goals and behaviours, most of which are extra-linguistic: we have basic physical desires, for things like food and sustenance; we have social goals and relationships; we have projects; and we create physical objects. Large language models simply aim to replicate human speech or writing. This means that their primary goal, insofar as they have one, is to produce human-like text. They do so by estimating the likelihood that a particular word will appear next, given the text that has come before.
The machine does this by constructing a massive statistical model, one which is based on large amounts of text, mostly taken from the internet. This is done with relatively little input from human researchers or the designers of the system; rather, the model is designed by constructing a large number of nodes, which act as probability functions for a word to appear in a text given its context and the text that has come before it. Rather than putting in these probability functions by hand, researchers feed the system large amounts of text and train it by having it make next-word predictions about this training data. They then give it positive or negative feedback depending on whether it predicts correctly. Given enough text, the machine can construct a statistical model giving the likelihood of the next word in a block of text all by itself.
AGI by 2027?
Fun with charts
JUN 05, 2024
Apparently OpenAI’s internal roadmap alleged that AGI would be achieved by 2027, according to the suddenly ubiquitous Leopold Aschenbrenner (recently fired by OpenAI, previous employed by SBF), who yesterday published the math to allegedly justify it:
I was going to write a Substack about how naive and problematic the graph was, but I am afraid somebody else has beaten me to it:
Well, ok I will add a few words:
GPT-4 is not actually equivalent to a smart high schooler. It can do some things better, some worse, very few reliably. Even it had access to a robot body (as is now common in some labs), I would not hire it to do most of things that high school students are often hired to do, like work as a lifeguard, work in restaurants, work in a grocery store, etc. Just because it can write average high school term papers based on massive numbers of samples doesn’t mean it’s on par with a smart high schoolers in their capacity to deal with the real world. Smart high schoolers often do original work, like competing for science fair prizes with original ideas or designing and implementing original computer systems singlehandedly. GPT-4 on its own ain’t doing any of that, as far as I know.
The double Y-axis makes no sense, and presupposes its own conclusion. The numbers on the left Y axis (“effective compute”) and their relation to the qualitative Y axis on the right (conflating model capabilities with human capabilities in a strange linear mishmosh) are arbitrary, verging on make-believe.
The graph assumes that all continued progress will be same — ignoring qualitatively unsolved problems (hallucinations, planning, reasoning etc), the fact that synthetic data may not be as useful as natural data, limits on then-possible energy distribution and compute, etc. Truly trillion pound baby stuff.
GPT-4 existed in August 2022. I can’t think of a single measure in which any existing model in 2024 outpace GPT-4 by as much as GPT-4 outpaced the state of the art in 2020. We are already behind the curve.
And honestly, every data point there is imaginary; we aren’t plotting real things here, no matter the legend might suggest.
All that said, Aschenbrenner does actually make and develop a very good (though not original) point in his new 165 page manuscript: we are (as Eliezer Yudkowsky has rightly argued for years) woefully underprepared for AGI whenever it comes. If you read his manuscript, please read it for his concerns about our underpreparedness, not for his sensationalist timelines.
The thing is, we should be worried, no matter how much time we have.
If the fiasco that has been GenAI has been any sign, self-regulation is a farce (Aschenbrenner’s former employer OpenAI apparently can’t even keep its own house in order), and the US legislature has made almost no progress thus far in reining in Silicon Valley in. It’s imperative that we do better.
Gary Marcus wrote his new book Taming Silicon Valley in part for this very reason.
Ilya Sutskever, OpenAI’s former chief scientist, launches new AI company
12:05 PM PDT • June 19, 2024
Ilya Sutskever, one of OpenAI’s co-founders, has launched a new company, Safe Superintelligence Inc. (SSI), just one month after formally leaving OpenAI.
Sutskever, who was OpenAI’s longtime chief scientist, founded SSI with former Y Combinator partner Daniel Gross and ex-OpenAI engineer Daniel Levy.
At OpenAI, Sutskever was integral to the company’s efforts to improve AI safety with the rise of “superintelligent” AI systems, an area he worked on alongside Jan Leike, who co-led OpenAI’s Superalignment team. Yet both Sutskever and then Leike left the company in May after a dramatic falling out with leadership at OpenAI over how to approach AI safety. Leike now heads a team at rival AI shop Anthropic.
Sutskever has been shining a light on the thornier aspects of AI safety for a long time now. In a blog post published in 2023, Sutskever, writing with Leike, predicted that AI with intelligence superior to humans could arrive within the decade — and that when it does, it won’t necessarily be benevolent, necessitating research into ways to control and restrict it.
He’s clearly as committed as ever to the cause today. Wednesday afternoon, a tweet announcing the formation of Sutskever’s new company states that: “SSI is our mission, our name, and our entire product roadmap, because it is our sole focus. Our team, investors, and business model are all aligned to achieve SSI. We approach safety and capabilities in tandem, as technical problems to be solved through revolutionary engineering and scientific breakthroughs.”
“We plan to advance capabilities as fast as possible while making sure our safety always remains ahead. This way, we can scale in peace. Our singular focus means no distraction by management overhead or product cycles, and our business model means safety, security, and progress are all insulated from short-term commercial pressures.”
Sutskever spoke with Bloomberg about the new company in greater detail, though he declined to discuss its funding situation or valuation.
The Series A Crunch Is No Joke
JUN 20, 2024
Welcome back to Cautious Optimism! It’s June 20th, 2024. CO was off yesterday due to a childcare crisis. Days off will occur as infrequently as possible, but family first — always. Let’s talk about the Series A crunch and Chinese venture capital! —
A venture stage “crunch” occurs when startups at one level of financing and development struggle as a group to reach the next level of financing, and, therefore, development.
Historically, the most common crunch discussed occurs at the Series A level, when startups that have raised Seed capital have a bear of a time securing their first lettered round. Recall that Seed-stage startups tend to be very nascent, revenue modest, and sharply unprofitable. They also tend to have quick growth.
Subscribed
The old rule of thumb was that startups growing in the triple-digits (year-over-year percentage) with $1 million worth of annual recurring revenue (ARR) or more could raise a Series A. Those days are long gone.
The bar is going up partly due to it being possible to raise multiple Seed rounds — Jason and I talked about this here on TWiST — and partially due to changing economic conditions making venture investors more conservative than before.
Here’s investor and AMEX denizen Trace Cohen on his portfolio:
Responses to this tweet brought up a litany of questions. With just the above information, folks argued, you can’t really say the companies in question deserve a Series A. What sort of net dollar retention are they sporting? Their customer value compared to their cost to acquire those customers? Their burn rate? Their burn multiple?
Those questions are all valid and are worth considering in any venture market — heady, moderate, or parsimonious. But what’s worth remembering is those concerns go away during boom times. Venture investors are willing to buy into the bull case for startups. In today’s stingy revenue multiple climate, private-market investors are taking a lot more into account than just quick growth and trailing revenue scale.
The argument used to go like this (back in, say, 2021):
Here’s a software startup doing $5 million in ARR today, growing at 300%. That’s $15 million worth of ARR next year. It’s not hard to chart a path for this company to $100 million worth of revenue; therefore, it will go public; and we should buy as much of it today as we can to reap the max reward later.
Now the argument looks like this:
Here’s a software company doing $5 million in ARR today, growing at 300%. Software growth rates have slowed, and capital availability has lessened. Therefore, this company’s growth will rapidly cool. And as many public software companies are earning sub-5x ARR multiples, and IPOs are rarer than pigs in tuxedos, the eventual return here is far from guaranteed. Therefore, we will limit our investment choices to only a few companies and offer them less capital at higher prices.
We’re discussing venture vibes here, but we can move from emotions to data if you want. SaaStr investor Jason Lemkin once told me the venture capital market is just the NASDAQ on steroids. He meant the higher the public market values of tech companies go, the more return private-market investors think they can make. More NASDAQ equals more venture capital investment.
If you want to go one step further, given how poorly growth-oriented stocks perform during periods of more expensive capital, the venture capital market is just the inverse of prevailing interest rates. When they are low, venture is high, and when they are high, venture is low.
Regardless of how far back you want to trace the line, venture vibes for non-AI companies are in the toilet. Enter Peter Walker from Carta with the data:
The dataset here ends in H1 2022 because you can’t expect Seed-stage startups to instantly raise their A. So we have to go back a bit to see older cohorts and how they have matured—or not.
Pour one out for Seed-stage biotech and SaaS companies that are performing the worst.
I doubt that the chart is as cruel for AI startups. This is why startups are racing to become AI companies even if they didn’t start life that way. Not only is it a gambit to garner customer attention, but it’s also a shot at getting more venture buy-in.
..More
The Series A Crunch or the Seedpocalypse of 2024
2012 was the year of the Seedpocalypse. Also called the Series A Crunch, a fear gripped Startupland : raising a Series A. Two years later, this indigestible excessive bolus of fundraising rounds hit the Series B market & Series Bs became the most challenging round to raise.
Whenever there are “too many” of fundraises of one type, the next round becomes the hardest to raise.
In 2024, the Series A Crunch has returned. Software companies that have achieved the previous era’s milestone, $1m or more in ARR, face a challenging Series A market. Why is this happening again?
Just as in 2012, a surge in seed investments met a relatively stable Series A market. The supply/demand imbalance creates a funding squeeze.
The orange crush of seed investment has outpaced the growth in Series A & Series B rounds. Many new seed funds started & the rate of company formation surged during the early 2020s driven by an ebullient capital markets.
Also, the definition of a Seed round has changed. The Seeds of the 2010 era are the pre-Seeds of today, making the comparison impure.
Regardless, Series As haven’t grown to nearly the extent of Seeds. During the last 14 years, the ratio of Seeds to Series As has grown from about 1.1 to 1, to 5 to 1. Meanwhile, the ratio of Bs to As is relatively constant : between 3 & 4 to 1.
With excess seed supply & in an era where forward public software multiples have reverted to the mean from their stratospheric levels, Series A rounds are harder to raise. AI startups, the darlings of the current era, are a notable exception. In this category, the heady multiples of 2021 & 2022 still apply.
But for classic SaaS companies, the Series A Crunch is real. In 18 months, the Series B will again become the hardest round to raise.
The Surgeon General Is Wrong. Social Media Doesn’t Need Warning Labels
Surgeon General Dr. Vivek H. Murthy is wrong about the harmful effects of social media on kids—but why let facts get in the way of a fabricated moral panic?
Mike Masnick
Updated Jun. 18, 2024 5:40PM EDT / Published Jun. 18, 2024 4:46AM EDT
Warning: Reading this article may cause you to question the Surgeon General’s reliance on feelings over science.
In 1982, then-U.S. Surgeon General Dr. C. Everett Koop said video games could be hazardous to children and warned of kids becoming “addicted” to them, causing problems for their “body and soul.”
This warning was not based on any actual science or evidence, but it kicked off decades of moral panic and fearmongering over the supposed risks of video games and children. This culminated in the Supreme Court rejecting a California law to require labeling of video games and restrict kids’ access to them, deeming it unconstitutional.
Studies have repeatedly debunked the claim that video games make kids more violent. Indeed, a recent Stanford meta-study of dozens of previous studies on kids and video games found no evidence of a connection between video games and violence. The researchers noted that if there was any correlation, it seemed to come from the public believing the unproven claims of a connection because politicians kept insisting it must be there.
History repeats itself.
U.S. Surgeon General Dr. Vivek H. Murthy has decided to call on Congress to put warning labels on social media sites, similar to those found on cigarettes. He claims this is necessary because “the mental health crisis among young people is an emergency—and social media has emerged as an important contributor.”
While Dr. Murthy admits at the very beginning of his plea that he does not have “perfect information,” he suggests that it’s important to use his “best judgment” and “act quickly.”
The major problem is that, as with Dr. Koop and video games, the evidence supports little more than the fact that politicians jumping on the moral panic bandwagon has resulted in many people falsely believing that social media is harmful to kids. Yes, there is a high-profile book out making many false claims about social media, but nearly all of the researchers in the space think it’s blown out of proportion.
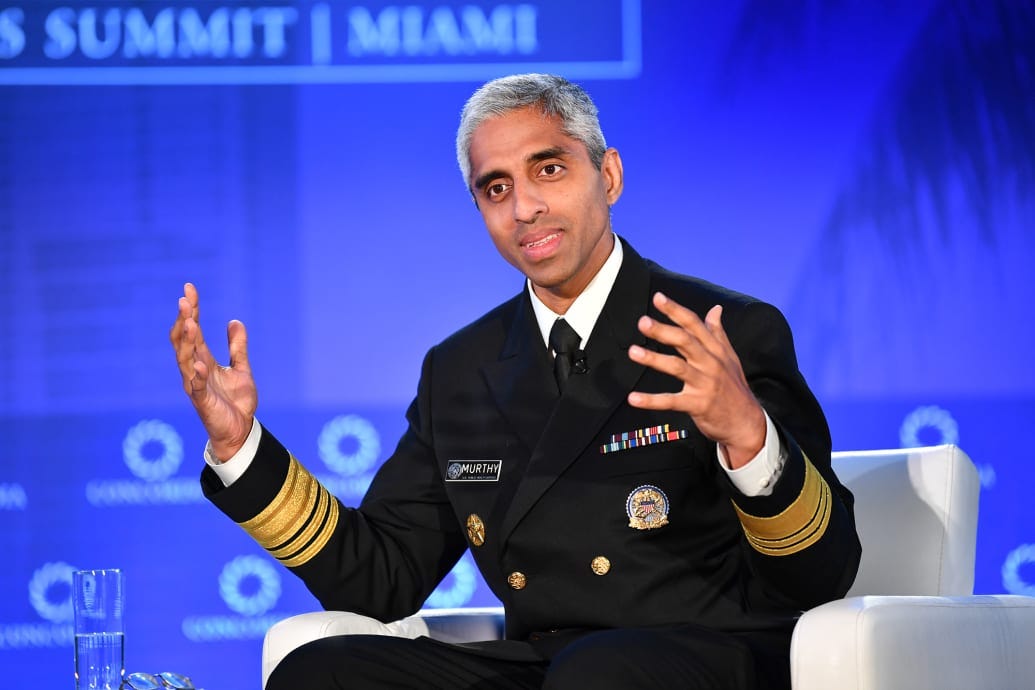
Jason Koerner/Getty Images for Concordia Summit
Dr. Candice Odgers, one of the world’s foremost experts in teen mental health and social media, has written extensively on how falling for this unproven moral panic is likely to cause more harm for teens, not less. This is in part because the correlation that does exist between teens and mental health is the result of teens seeking out social media in response to a lack of access to mental health support.
One needs to go no further than Murthy’s own (widely misrepresented) report last year on social media and youth mental health to see that the evidence regarding teen mental health and social media is way more complex and nuanced than Murthy now makes it out to be. Murthy’s own report stated: “A majority of adolescents report that social media helps them feel more accepted (58 percent), like they have people who can support them through tough times (67 percent), like they have a place to show their creative side (71 percent), and more connected to what’s going on in their friends’ lives (80 percent). In addition, research suggests that social media-based and other digitally-based mental health interventions may also be helpful for some children and adolescents by promoting help-seeking behaviors and serving as a gateway to initiating mental health care.”
Indeed, his own report explicitly highlights how helpful social media has been for the mental well-being of “lesbian, gay, bisexual, asexual, transgender, queer, intersex and other youths” because it enables “peer connection, identity development and management, and social support.”
That seems like a strange thing to require a “Surgeon General’s Warning” about.
Video of the Week
Danny Rimer of Index Ventures: The Biggest Lessons from Missing Snap, Airbnb, Spotify and Facebook
AI of the Week
Anthropic has a fast new AI model — and a clever new way to interact with chatbots
/
Claude 3.5 Sonnet is apparently Anthropic’s smartest, fastest, and most personable model yet.
By David Pierce, editor-at-large and Vergecast co-host with over a decade of experience covering consumer tech. Previously, at Protocol, The Wall Street Journal, and Wired.
Jun 20, 2024, 7:00 AM PDT
Image: Anthropic
The AI arms race continues apace: Anthropic is launching its newest model, called Claude 3.5 Sonnet, which it says can equal or better OpenAI’s GPT-4o or Google’s Gemini across a wide variety of tasks. The new model is already available to Claude users on the web and on iOS, and Anthropic is making it available to developers as well.
Claude 3.5 Sonnet will ultimately be the middle model in the lineup — Anthropic uses the name Haiku for its smallest model, Sonnet for the mainstream middle option, and Opus for its highest-end model. (The names are weird, but every AI company seems to be naming things in their own special weird ways, so we’ll let it slide.) But the company says 3.5 Sonnet outperforms 3 Opus, and its benchmarks show it does so by a pretty wide margin. The new model is also apparently twice as fast as the previous one, which might be an even bigger deal.
AI model benchmarks should always be taken with a grain of salt; there are a lot of them, it’s easy to pick and choose the ones that make you look good, and the models and products are changing so fast that nobody seems to have a lead for very long. That said, Claude 3.5 Sonnet does look impressive: it outscored GPT-4o, Gemini 1.5 Pro, and Meta’s Llama 3 400B in seven of nine overall benchmarks and four out of five vision benchmarks. Again, don’t read too much into that, but it does seem that Anthropic has built a legitimate competitor in this space.
Claude 3.5’s benchmark scores do look impressive — but these things change so fast.
Image: Anthropic
What does all that actually amount to? Anthropic says Claude 3.5 Sonnet will be far better at writing and translating code, handling multistep workflows, interpreting charts and graphs, and transcribing text from images. This new and improved Claude is also apparently better at understanding humor and can write in a much more human way.
Along with the new model, Anthropic is also introducing a new feature called Artifacts. With Artifacts, you’ll be able to see and interact with the results of your Claude requests: if you ask the model to design something for you, it can now show you what it looks like and let you edit it right in the app. If Claude writes you an email, you can edit the email in the Claude app instead of having to copy it to a text editor. It’s a small feature, but a clever one — these AI tools need to become more than simple chatbots, and features like Artifacts just give the app more to do.
The new Artifacts feature is a hint at what a post-chatbot Claude might look like.
Image: Anthropic
Artifacts actually seems to be a signal of the long-term vision for Claude. Anthropic has long said it is mostly focused on businesses (even as it hires consumer tech folks like Instagram co-founder Mike Krieger) and said in its press release announcing Claude 3.5 Sonnet that it plans to turn Claude into a tool for companies to “securely centralize their knowledge, documents, and ongoing work in one shared space.” That sounds more like Notion or Slack than ChatGPT, with Anthropic’s models at the center of the whole system.
For now, though, the model is the big news. And the pace of improvement here is wild to watch:
..More
Nvidia’s Ascent to Most Valuable Company Has Echoes of Dot-Com Boom
Chip maker passes Microsoft for top spot, just as John Chambers-led Cisco Systems did two decades ago. He says the situation now is different.
Nvidia’s chips have been the workhorses of the AI boom. IAN MAULE/BLOOMBERG NEWS
By Asa Fitch
Nvidia became the world’s most valuable listed company Tuesday thanks to the demand for its artificial-intelligence chips, leading a tech boom that brings back memories from around the start of this century.
Nvidia’s chips have been the workhorses of the AI boom, essential tools in the creation of sophisticated AI systems that have captured the public’s imagination with their ability to produce cogent text, images and audio with minimal prompting.
The last time a big provider of computing infrastructure was the most valuable U.S. company was in March 2000, when networking-equipment company Cisco took that spot at the height of the dot-com boom.
Cisco was riding the wave of a different revolution—the internet—where its products powered that budding industry. Like Nvidia, Cisco also surpassed Microsoft to become the most valuable company.
Nvidia CEO Jensen Huang says the company is building ‘AI factories’ that take in data and churn out intelligence. PHOTO: IAN MAULE/BLOOMBERG NEWS
John Chambers, who was chief executive of Cisco during the dot-com boom, said there are some parallels, but the dynamics of the AI revolution are different from previous ones such as the internet and cloud computing. Chambers, now a venture investor, has made big bets on AI in cybersecurity and other arenas.
“The implications in terms of the size of the market opportunity is that of the internet and cloud computing combined,” he said. “The speed of change is different, the size of the market is different, the stage when the most valuable company was reached is different.”
Nvidia, a 31-year-old company, became the world’s most valuable listed firm on Tuesday. The stock closed at $135.58, giving the chip maker a valuation of $3.335 trillion, just above Microsoft at $3.317 trillion.
It marks the first time a company other than Microsoft or Apple has held the title of largest company since February 2019, when Amazon.com briefly topped the list. Nvidia was ranked fifth largest by market valuation a year ago and was ranked 10th largest two years ago. Five years ago, it wasn’t in the top 20 largest companies.
The scramble among tech giants such as Microsoft, Meta and Amazon to lead the way in AI’s development and capture its hoped-for benefits has led to a chip-buying spree that lifted Nvidia’s revenue to unprecedented heights. In its latest quarter, the company brought in $26 billion, more than triple the same period a year before.
Nvidia’s stock was the best performer in the S&P 500 in 2023 and has more than tripled in value over the past 12 months. The company’s value hit $3 trillion this month, less than four months after it reached the $2 trillion mark.
Nvidia split its shares 10-for-1 this month, a move aimed at lowering the price of each share and making it more accessible to investors.
The stunning rise has won plaudits from analysts who agree with Chief Executive Jensen Huang’s assertion that AI is the foundation of a new industrial revolution to which the company is the key supplier. Huang says Nvidia is building “AI factories” that take in data and churn out intelligence.
Nvidia “will be the most important company to our civilization over the next decade as the world becomes more AI-driven,” CFRA Research analyst Angelo Zino said recently. The chips Nvidia pioneered will be the most important invention of this century, he said.
The Expanding Universe of Generative Models
Speakers: Yann LeCun, Nicholas Thompson, Kai-Fu Lee, Daphne Koller, Andrew Ng, Aidan Gomez
January 16, 202415:00–15:45 CET
Generative AI is advancing exponentially. What is happening at the frontier of research and application and how are novel techniques and approaches changing the risks and opportunities linked to frontier, generative AI models?
DeepMind’s new AI generates soundtracks and dialogue for videos
11:03 AM PDT • June 17, 2024
DeepMind, Google’s AI research lab, says it’s developing AI tech to generate soundtracks for videos.
In a post on its official blog, DeepMind says that it sees the tech, V2A (short for “video-to-audio”), as an essential piece of the AI-generated media puzzle. While plenty of orgs, including DeepMind, have developed video-generating AI models, these models can’t create sound effects to sync with the videos that they generate.
“Video generation models are advancing at an incredible pace, but many current systems can only generate silent output,” DeepMind writes. “V2A technology [could] become a promising approach for bringing generated movies to life.”
DeepMind’s V2A tech takes the description of a soundtrack (e.g. “jellyfish pulsating under water, marine life, ocean”) paired with a video to create music, sound effects and even dialogue that matches the characters and tone of the video, watermarked by DeepMind’s deepfakes-combating SynthID technology. The AI model powering V2A, a diffusion model, was trained on a combination of sounds and dialogue transcripts as well as video clips, DeepMind says.
“By training on video, audio and the additional annotations, our technology learns to associate specific audio events with various visual scenes, while responding to the information provided in the annotations or transcripts,” according to DeepMind.
Mum’s the word on whether any of the training data was copyrighted — and whether the data’s creators were informed of DeepMind’s work. We’ve reached out to DeepMind for clarification and will update this post if we hear back.
AI-powered sound-generating tools aren’t novel. Startup Stability AI released one just last week, and ElevenLabs launched one in May. Nor are models to create video sound effects. A Microsoft project can generate talking and singing videos from a still image, and platforms like Pika and GenreX have trained models to take a video and make a best guess at what music or effects are appropriate in a given scene.
..More
News Of the Week
Apple Suspends Work on Next Vision Pro, Focused on Releasing Cheaper Model in Late 2025
By Wayne Ma and Qianer Liu
June 18, 2024, 8:00am PDT
Apple has told at least one supplier that it has suspended work on its next high-end Vision headset, an employee at a manufacturer that makes key components for the Vision Pro said. The pullback comes as analysts and supply chain partners have flagged slowing sales of the $3,500 device.
The company is still working on releasing a more affordable Vision product with fewer features before the end of 2025, the person involved in its supply chain and a person involved in the manufacturing of the headsets said. Apple originally planned to divide its Vision line into two models, similar to the standard and Pro versions of the iPhone, according to people involved in its supply chain and former Apple employees who worked on the devices.
The Takeaway
Apple is suspending work on its next high-end Vision headset as sales of the $3,500 Vision Pro slow, focusing instead on releasing a cheaper model by the end of 2025. The shift could spark a similar pullback by competitors building expensive headsets.
Apple’s decision to halt work on the next version of its high-end headset is the latest example of the company reshuffling priorities. Apple has ramped up work on AI-powered features while paring back money-losing projects like its self-driving car, which it canceled earlier this year after spending nearly a decade on development. Augmented reality is one of Apple’s biggest bets. The company aims to eventually replace the iPhone with lightweight glasses, and the Vision Pro is the first step in building consumer and developer interest in that effort.
Although it’s possible that Apple could resume work on a high-end Vision product down the road, suspending development of the next high-end headset for now could have repercussions for the rest of the AR and virtual reality industry, which views the headset as a litmus test of consumer appetite for a premium device. Meta Platforms, for instance, started work in November on a new high-end headset, internally code-named La Jolla, according to multiple current and former Meta employees, five months after Apple announced the Vision Pro. Before that, in January 2023, Meta had axed plans to build a more expensive Quest headset after it saw weak sales of its $1,500 Quest Pro. A Meta spokesperson didn’t reply to a request for comment.
An Apple spokesperson declined to comment.
Is the news industry ready for another pivot to video?
Aggregate data from 47 countries shows all the growth in platform news use coming from video or video-led networks.
By NIC NEWMAN June 20, 2024, 11:37 a.m.
Seven or eight years ago much of the news industry lost its head over a supposed “pivot to video” after Facebook pushed live and other formats with the promise of monetization to come. That didn’t work out so well for publishers, many of whom had hired expensive video teams and found they had to row back after interest waned.
But now social platforms are going “all-in” on video again as they increasingly compete with each other for attention in a more fragmented and uncertain landscape. Platforms like TikTok have revitalized shorter formats, forcing YouTube and Meta to radically change their own product-sets, while X (Twitter) is apparently now a video-first network focussing on incentivizing creators and making it harder for publishers to drive referrals back to their news sites.
The Digital News Report 2024 from the Reuters Institute for the Study of Journalism, published this week, documents the impact of some of these changes on audience consumption and finds that, this time around, [whisper it] the shift to video may be more lasting and more significant than many expect.
Aggregate data from 47 countries shows all the growth in platform news use coming from video or video-led networks such as YouTube, TikTok, and Instagram while legacy networks such as Facebook are becoming less important. This is particularly the case in countries outside the U.S. and Europe, where video consumption is growing fastest amongst young populations as data charges fall. YouTube is used for news by almost 31% of our global sample weekly, and in countries such as India and South Africa around half our survey sample say use the platform for news.
..More
Cerebras, an Nvidia Challenger, Files for IPO Confidentially
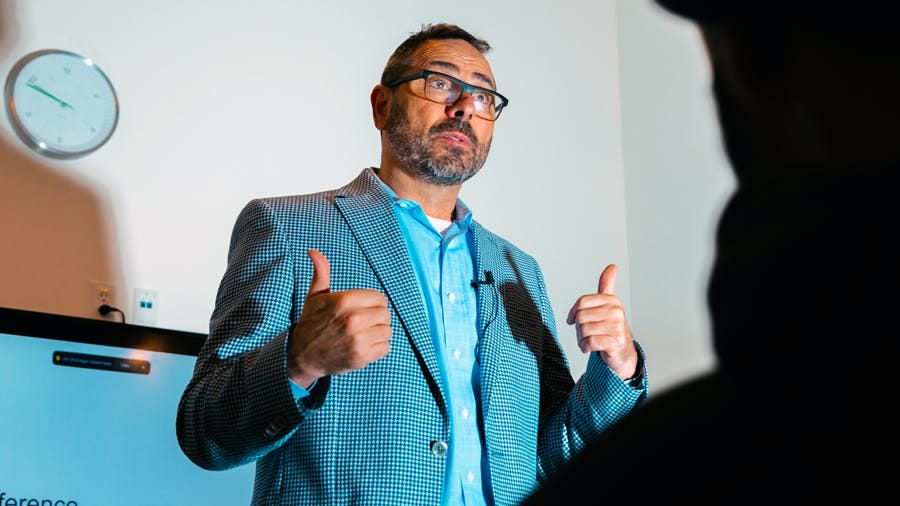
By Natasha Mascarenhas and Stephanie Palazzolo
Jun 20, 2024, 4:08pm PDT
Cerebras, a developer of artificial intelligence chips that’s trying to wrestle business from Nvidia, has filed confidentially with securities regulators for an initial public offering, according to a person involved in the decision.
The startup has also notified Delaware, the state in which it is incorporated, that it is creating preferred shares priced at a deep discount to its last private funding round. The move could make its shares more attractive to private investors ahead of an IPO and those who participate in the public listing.
The Takeaway
Cerebras, which is developing AI chips to compete with giant Nvidia, has filed plans for a public offering at the same time it slashed its share price.
The IPO plans show the eight-year-old company wants to ride a wave of investor enthusiasm over AI hardware sales that have made Nvidia the world’s most valuable company and boosted scores of other stocks. Cerebras will need to show how it plans to get a piece of the market for AI server chips and loosen Nvidia’s grip on it.
The startup’s financial results couldn’t be learned. It said in a blog post in December that it had recently reached “cash flow break-even,” without elaborating. Cerebras declined to comment.
In early June, Cerebras authorized a share price of $14.66 for around 27 million of new shares, according to a filing shared by Prime Unicorn Index. That is sharply lower than the $27.74 share price from its 2021 Series F funding round that valued it at over $4 billion. The new share authorization suggests Cerebras is valuing itself at around $2.5 billion.
It’s not clear whether Cerebras has officially sold the shares or who will own them. It's common for companies to raise capital ahead of an IPO, sometimes to shore up their balance sheet before public investors consider buying the stock.
..More
Startup of the Week
Final Cut Camera and iPad Mulitcam are Truly Revolutionary
The potential of Apple's latest filmmaking tool is sure to change the game.
Apple
Jun 20, 2024
Way back in 1999 I remember clearly telling a friend "you know, eventually video cameras will just be a credit card with a screen on one side and a camera on the other, and it'll fly."
We're not quite there (drones come close), but I thought about that conversation for the first time in 25 years when testing out the new Final Cut Camera and iPad Final Cut Multicam features that are rolling out today. We really are in a "the future is here now" moment.
Lots of folks have camera apps, of course (we have been shooting with the Blackmagic app a lot lately ourselves). And multi-cam on an iPad is something a few others have tried already. But Apple is pulling their signature trick here once more and made it "just work" in a way nobody else has managed to achieve.
What is the Update, Exactly?
Apple
It's this simple: you install Final Cut Camera on your phone and you get a dynamite camera app. Then you fire up Final Cut Pro on your iPad, create a multi-camera project, and search for and pair phones that have Final Cut Camera installed. They all come up as sources on your iPad, which can navigate to one source.
From there you can simply work in multicam editing with ease. You can directly record (now with a ton of storage options thanks to USB-C), or you can livestream it to a variety of platforms automatically.
But here's the key: it works. Each phone records full resolution video locally, and only streams a proxy to the iPad. Then, after you are done shooting, all the full resolution video syncs in the background and creates a multicam project of full resolution video you can keep editing later.
You can do a live edit, then keep tweaking it after the fact. It works in proxy, and then gives you full res for your final. And it does it all wirelessly.
..More
Share this post