A reminder for new readers. That Was The Week includes a collection of my selected readings on critical issues in tech, startups, and venture capital. I selected the articles because they are of interest to me. The selections often include things I entirely disagree with. But they express common opinions, or they provoke me to think. The articles are sometimes long snippets to convey why they are of interest. Click on the headline, contents link or the ‘More’ link at the bottom of each piece to go to the original. I express my point of view in the editorial and the weekly video below.
Congratulations to this week’s chosen creators: @candice_odgers, @cdallarivamusic, @rex_woodbury,
Contents
Editorial: When is a Bubble not a Bubble?
New Apple Vision Pro Personas
Mike Maples on Y Combinator
Editorial
I’ve taken to writing this on Friday morning. I put the curated content together Thursday evening, which gives me overnight to reflect. Usually, the title comes first and is somehow correlated to the content below.
This week, there is a lot about AI. The Y Combinator story in AI of the week is the story that the “bubble” will be challenged due to a lack of training data. In contrast, the story is that AI will remove so many jobs that the larger companies have formed a consortium to allay fears.
I also created a new section separating out Venture Capital. This is the week of quarterly updates from Q1. They suggest there is no bubble at all. Only Amazon’s multi-billion dollar investment in Anthropic stands out.
But for me, the question posed in the title is - When is a Bubble not a Bubble? - is not triggered by the AI stories. The Economist’s Simon Cox writes about China and its future in a newsletter and the linked article. He frames it well:
In 2006, for example, China’s leaders declared the need to “rely more than ever on scientific and technological progress and innovation to drive a qualitative leap in productivity”. Science and technology, they added, are “the concentrated embodiment…of advanced productive forces”. That ambition, and indeed that diction, sound very similar to the slogans emanating from Beijing today. Xi Jinping, China’s leader, has, for example, urged provincial governments to cultivate “new productive forces”, based on science and technology. In this week’s issue I explore what those words might mean.
As Simon points out, “productive forces” is a formulation derived from Hegel and Marx. It combines technology and human beings into a duality that expresses how we produce things. Indeed, there is no pure “technology” separate from human beings and the division of labor. Productivity is the expression of both and the measurable thing.
In the Western enlightenment tradition, we use the word progress to mean the same thing.
All progress requires humans to invent time-saving methods to reduce the effort involved in making and doing things.
China’s discussion (especially if you remove the word China) is about building the future through innovation. It stands in contrast to the dominant discussions here in the US - Regulation, the dangers of Social Media, Immigration, Women’s Right to Choose, Guns, and even Climate. And a lot of pessimism around technology and science.
That is except for in the startup ecosystem. The dominant Silicon Valley belief system is similar to Simon Cox’s description of China’s goals.
Accelerated Innovation dominates the set of assumptions in the Bay Area. Why? Because AI, Nuclear Fusion, Decentralized Networks, Global Ambition, and the skills and money they require all live here. And their potential is real. And the timing of the potential is near-term (several years).
Strangely, the US Government seems to consider innovation, especially “Big Tech,” a problem. China and Silicon Valley seem to consider it a solution. And by “Silicon Valley,” I do not only mean geographically but also as a way of thinking.
That bifurcation of optimism and pessimism, enshrined in a Government that wants to restrict tech company power, has led many in the Valley to abandon traditional two-party politics and increasingly articulate agendas that are both optimistic and independent of Government. Government is perceived as a cost of doing business, not a benefit.
So, the innovation that comes out of Silicon Valley and the money it attracts are often scorned by those who are not part of it. The word “Bubble” is heavily laden and used to imply that there is nothing valid, real, or transformational. The money is simply irrational.
“Bubble” is a pessimists word for “fake”.
It goes alongside other narratives that cast doubt on innovation. In some ways, Tomasz Tunguz's piece on the shrinking attention span implies a problem caused by the abundance of content and limited time to read it. Although one might consider the ability to parse information and determine whether it is attention-worthy and do it quickly would be a good thing.
The idea that teens commit suicide and get depressed due to alienating social media comes to mind as another anti-technology narrative. The first ‘Essay of the Week’ from Nature magazine presents a strong case that this is bogus.
Rex Woodbury’s “Weapons of Mass Production” and Michael Spencer and Chris Dalla Riva’s “AI and the Future of Music Production and Creation” (The Day the Music Lied) point to the explosion of production and creative production that AI will trigger.
Rex:
Spotify reinvented music distribution. It put 100 million songs in your pocket. Generative AI will reinvent music production. There are a number of early-stage startups that let you toggle artist, genre, and ~vibe~ to create a wholly new work—e.g., “Create a Miley Cyrus breakup song with a sad, wistful feeling to it.” Of course, these companies will need to navigate the labyrinth of music rights, but some version of these tools feels inevitable.
This example embodies a broader shift we’re seeing from distribution ➡️ production.
Michael Spencer and Chris Dalla Riva:
In summary, the music industry will likely come to embrace much of this technology as long as AI firms properly license the music catalogs necessary to train their models. This still begs one final question: Is any of this good for music?
It’s important to unpack words like Bubble. They live in a context. As Simon Cox discusses, the future depends on progress, innovation, or “productive forces.” So, this “Bubble” is not a bubble.
Essays of the Week
The great rewiring: is social media really behind an epidemic of teenage mental illness?
The evidence is equivocal on whether screen time is to blame for rising levels of teen depression and anxiety — and rising hysteria could distract us from tackling the real causes.
The Anxious Generation: How the Great Rewiring of Childhood is Causing an Epidemic of Mental Illness Jonathan Haidt Allen Lane (2024)
Two things need to be said after reading The Anxious Generation. First, this book is going to sell a lot of copies, because Jonathan Haidt is telling a scary story about children’s development that many parents are primed to believe. Second, the book’s repeated suggestion that digital technologies are rewiring our children’s brains and causing an epidemic of mental illness is not supported by science. Worse, the bold proposal that social media is to blame might distract us from effectively responding to the real causes of the current mental-health crisis in young people.
Haidt asserts that the great rewiring of children’s brains has taken place by “designing a firehose of addictive content that entered through kids’ eyes and ears”. And that “by displacing physical play and in-person socializing, these companies have rewired childhood and changed human development on an almost unimaginable scale”. Such serious claims require serious evidence.
Collection: Promoting youth mental health
Haidt supplies graphs throughout the book showing that digital-technology use and adolescent mental-health problems are rising together. On the first day of the graduate statistics class I teach, I draw similar lines on a board that seem to connect two disparate phenomena, and ask the students what they think is happening. Within minutes, the students usually begin telling elaborate stories about how the two phenomena are related, even describing how one could cause the other. The plots presented throughout this book will be useful in teaching my students the fundamentals of causal inference, and how to avoid making up stories by simply looking at trend lines.
Hundreds of researchers, myself included, have searched for the kind of large effects suggested by Haidt. Our efforts have produced a mix of no, small and mixed associations. Most data are correlative. When associations over time are found, they suggest not that social-media use predicts or causes depression, but that young people who already have mental-health problems use such platforms more often or in different ways from their healthy peers1.
These are not just our data or my opinion. Several meta-analyses and systematic reviews converge on the same message2–5. An analysis done in 72 countries shows no consistent or measurable associations between well-being and the roll-out of social media globally6. Moreover, findings from the Adolescent Brain Cognitive Development study, the largest long-term study of adolescent brain development in the United States, has found no evidence of drastic changes associated with digital-technology use7. Haidt, a social psychologist at New York University, is a gifted storyteller, but his tale is currently one searching for evidence.
..More
The Day the Music Lied
Chris Dalla Riva, February-March, 2024.
When the song “Heart on My Sleeve” by Drake & The Weeknd came out in April 2023, it shook the music industry. The industry wasn’t shaken because two of its biggest stars were collaborating. It was shaken because the two pop stars weren’t collaborating.
“Heart on My Sleeve” was created by a shadowy figure named Ghostwriter, the song getting millions of streams across TikTok, YouTube, and Spotify before Universal Music Group forced platforms to take it down. Ghostwriter used new technology powered by artificial intelligence to closely simulate the voices of both Drake and The Weeknd. Despite the questionable ethics behind the creation of the song, listeners loved it. Here are some posts on Twitter from around the time the song went viral.
Daily Loud: “this Drake & The Weeknd A.I song is a hit”
@MeekMill: “A.I generated song Drake ft. The Weeknd - heart on my sleeve via @YouTube this my 5th time banging this and it’s flame…. We need new music from y’all 2”
@TrungTPhan: “This song is a new collaboration between Drake and The Weeknd. It’s called “Heart On My Sleeve” and is an absolute banger. It’s also completely AI generated.”
@itroll93: “Heart on my Sleeve is so much better than anything Drake has put out in two years”
Technological upheaval always seems to affect music before other cultural industries. When the file-sharing site Napster was forced to shut down in 2001, for example, Netflix was still a private company mailing DVDs and six years away from launching a video streaming service. In fact, in the same time frame where you went from buying a VHS to buying a DVD in order to watch a movie at home, you also bought vinyl, 8-tracks, cassettes, CDs, MP3s, and a few other things to listen to music at home.
Part of the reason that musical technology is always evolving is due to cost. Making music is dramatically cheaper than making movies. It’s also due to size and complexity. Downloading a song to my phone only takes up about 3 megabytes of space. A television episode is closer to 100 megabytes and a movie is at least 700.
Because of these factors, along with a few others, artificial intelligence technology will likely disrupt the music industry much sooner than other cultural industries, “Heart on My Sleeve” merely a harbinger of what is to come. But to understand what “Heart on My Sleeve” is a harbinger of, we need to understand how the music industry itself has changed in the last few decades.
The Changing Incentives in the Music Industry
What we think of as the modern music industry didn’t exist until the late-1800s when Thomas Edison invented the phonograph. Before that, the music industry was largely focused around sheet music sales and live performance. The phonograph and subsequent improvements made it so that music could be recorded and sold to the public to play again and again. From that point forward, the music industry was fundamentally a product industry, selling music in many different formats to consumers, along with devices to play that music on.
Because of this, the earliest and most successful music labels were partially technology companies. Edison Records invented the wax cylinder. RCA Victor invented the Victrola. Columbia Records invented the LP. Decca Records invented stereo sound. Philips Records invented the cassette. Sony invented the CD. If we look at industry revenues over the past half century, we can see that the products – usually internally invented – that powered the music industry.
Everything changed around the year 2000, though. That’s when industry revenues began to collapse as digital file sharing sites like Napster, Limewire, and Kazaa ballooned in popularity. File sharing and the technology that made it possible, including the world wide web and the MP3, represented a break with the past because they were a disruptive technology that emerged outside of the music industry. The industry’s response to this disruption was twofold.
Weapons of Mass Production
What the Industrial Revolution Was to Physical Production, the AI Revolution Is to Digital Production
APR 03, 2024
Post Malone is having a good month.
The artist was featured on Beyoncé’s new album Cowboy Carter in the song “LEVII’S JEANS.” And in a few weeks, Post Malone will feature again on spring’s other big release—Taylor Swift’s The Tortured Poets Department.
Post Malone’s feature on Tortured Poets comes in a song called “Fortnight,” and the song already leaked online. Well, not actually—but a lot of people were fooled into thinking so. An AI-generated version of “Fortnight” took TikTok by storm last month (it’s actually a banger) and duped everyone into believing the track leaked.
This is the world of AI: a song can be entirely generated—fabricated—but be plausibly, passably excellent. (The same thing happened with Swift’s track “Suburban Legends” last fall before the 1989 (Taylor’s Version) release, and many fans ended up preferring the AI version.)
Spotify reinvented music distribution. It put 100 million songs in your pocket. Generative AI will reinvent music production. There are a number of early-stage startups that let you toggle artist, genre, and ~vibe~ to create a wholly new work—e.g., “Create a Miley Cyrus breakup song with a sad, wistful feeling to it.” Of course, these companies will need to navigate the labyrinth of music rights, but some version of these tools feels inevitable.
This example embodies a broader shift we’re seeing from distribution ➡️ production.
Distribution vs. Production
The internet was a distribution revolution.
At its simplest, the internet is all about networks. You can even visualize those networks. Back in the 2000s, a man named Barrett Lyon used traceroutes (maps of how data travels online from its source to its destination) to create an image that showed internet activity circa 2003:
In 2021, Lyon updated his visualization. This time, rather than using traceroutes, he used Border Gateway Protocol routing tables to get a more accurate view. The updated image is composed of clusters of network regions—examples of clusters include the U.S. Department of Defense’s Non-Classified Internet Protocol Network, Shenzhen Tencent Computer Systems, and Amazon’s AWS. (You can watch a video of the visualization here.)
The internet is all about rails—rails for information, data, content, commerce, and communication 🛤️.
While the internet was a distribution revolution, AI is a production revolution. Generative AI makes it really, really easy to make stuff. It blows open the floodgates of production.
We’ve seen past technology eras for both distribution and production. The automobile was largely a distribution revolution—people could travel easily across long distances, which led to sprawling cities and offshoot industries like the shopping mall and the credit card. Products could also travel easily, giving way to the trucking industry and so on.
The Industrial Revolution, meanwhile, was a production revolution. Goods could be manufactured cheaply at scale. This paved the wave for the automobile revolution, of course; cars could be mass produced because of innovations developed during the Industrial Revolution (and later Henry Ford’s assembly line).
This time around, things are happening in reverse. We already had the distribution revolution with the internet; we have the rails for digital distribution. Now we’re getting mass digital production with AI.
This means we’re going to see an explosion in production tools.
The Tools of Production
The steam engine emerged in the early 1700s and mechanized factory production. All of a sudden factories didn’t need to be located on or near sources of water power. Manufacturing exploded.
What’s the digital equivalent of the steam engine? Arguably, the transformer model. Foundation models are the building blocks for a new era of production. Factories succeeded with economies of scale and with specialized production: you got really good at making buttons in this factory; you got really good at making wheels in that factory. It will be the same with models, which can be fine-tuned and trained for specific use cases—for legal briefs, for tax filings, for movies, for coding.
Some of the most exciting startups right now are startups that power digital production. In seconds, I can write this prompt in Midjourney and get beautiful artwork for this Digital Native piece:
“We are in a factory and there is an enormous conveyer belt powered by big machines. On the conveyor belt are a series of digital objects being produced. There are robots working at the conveyor belt. The vibe of the image is sleek and polished and futuristic. The style is cyberpunk. High-resolution, 4K.”
That would take a human artist hours to create.
It’s the same for video. Last month’s The Future Is a Dupe dug into OpenAI’s Sora, an AI model that can create stunning videos from text prompts. Here’s the video output for the prompt:
China’s future economy
Simon Cox
China economics editor
In 2007 I wrote a special report about the technological ambitions of China and India. It feels like a long time ago. The iPhone, unveiled only months before, was still rare. The most envied chipmaker was Intel. The best known Chinese tech brand was probably Lenovo, which had bought IBM’s laptop business a few years earlier. The technological division of labour between China and America was far less fraught. A junior reporter like myself could don disposable overshoes and take a peek inside the fabs of Semiconductor Manufacturing International Corporation (SMIC) in Shanghai. He could also get an interview with Jack Ma.
But some things from that era seem strikingly familiar. In 2006, for example, China’s leaders declared the need to “rely more than ever on scientific and technological progress and innovation to drive a qualitative leap in productivity”. Science and technology, they added, are “the concentrated embodiment…of advanced productive forces”. That ambition, and indeed that diction, sound very similar to the slogans emanating from Beijing today. Xi Jinping, China’s leader, has, for example, urged provincial governments to cultivate “new productive forces”, based on science and technology. In this week’s issue I explore what those words might mean.
The concept remains fuzzy, perhaps deliberately so. For some, “new productive forces” hark back to the dialectics of Georg Hegel, a 19th-century German philosopher who inspired Karl Marx. For others, the term refers to the mainstream economic concept of “total factor productivity”, which measures output that cannot be attributed to measurable inputs, such as labour and capital. Some analysts associate the phrase with futuristic technologies such as brain-computer interfaces, Mars exploration and lithographic cannons (a new way to etch circuitry onto chips). Others have humbler visions. One official at a prominent state-owned distillery recently argued that the new productive forces can be found in hard spirits.
China’s productive forces have of course grown considerably since 2006. And the country can boast some remarkable feats of technological progress. China now ranks 12th on the Global Innovation Index, which blends about 80 indicators, far above the rank you would expect for a country with its income per person.
But there are two ways to look at China’s outlying position. One conclusion is that its technological capabilities far outstrip its modest level of economic development. A more sombre conclusion is that its economy is surprisingly poor, given the country’s technological sophistication. If technology is “the lever of riches”, as Joel Mokyr, an economic historian, has argued, why is China getting so little leverage from its labs and fabs?
There is always a lag between the creation of a new technology, its widespread adoption by firms and its impact on productivity. Some scholars think adoption and diffusion has been slow in China. Jeffrey Ding of George Washington University has looked separately at some of the diffusion-related indicators feeding into the overall Global Innovation Index. On these measures, China’s rank was still in the 40s in 2020, he argues. In its pursuit of a “qualitative leap in productivity”, China’s economy perhaps ought to rely more on scientific and technological diffusion rather than invention. My 2007 special report argued much the same thing. Perhaps that era was not so different after all.
..More
This Message Will Self-Destruct in 33 Seconds
The average American attention span has fallen from 150 seconds in 2004 to 75 seconds in 2012 to 47 seconds in 2023 - a 5-6% annual rate of decline.
How does this compare to these blog posts?
In 2013, the average reader dwelled on this site for 47 seconds.
Today, it’s 33 seconds, a 3.6% decline - which is a bit better ! but probably within the realm of statistical noise
Over the past decade, the average word count per post on this site has fallen 40% from approximately 560 at the peak to approximately 350 today.
So the deflation is consistent. We could argue the posts themselves are shorter, which reduces dwell time - but it’s the other way around.
Content on the internet has compressed : YouTube shorts & tweets come to mind. They provide a faster time to value/dopamine. Authors, rewarded for shorter content, mirror the rest of the web.
..More
1 in 6 People Will Be Aged 65+ by 2050
Overview
What we’re showing
The size of the world’s population aged 65+, for 1980, 2021, and 2050 (projected).
Global aging
The size of the world’s senior population isn’t just growing in absolute numbers, it’s also growing as a share of the overall total. For example, in 2021, 1 in 10 people worldwide were over the age of 65. By 2050, this is likely to be around 1 in 6.
Data sources
https://www.un.org/development/desa/pd/content/launch-world-social-report-2023
Venture Investing this Week
Global Venture Funding In Q1 2024 Shows Startup Investors Remain Cautious
April 4, 2024
The first quarter of 2024 was the second-lowest on record for global startup funding since the beginning of 2018, according to an analysis of Crunchbase data.
Global venture funding reached $66 billion in the first quarter, up 6% quarter over quarter but down 20% year over year, Crunchbase data shows.
The lowest in the past six years was the previous quarter, Q4 2023. While funding amounts for the most recent quarter will increase a bit over time as data is added and reviewed, the overall investor outlook remains cautious.
Table of Contents
Healthcare and AI lead
AI continued to stand out as a leading sector for investment in the first quarter. Companies in the AI sector raised $11.4 billion in Q1, or around 17% of global funding, Crunchbase data shows. The largest rounds went to:
Beijing-based Moonshot AI, a large language model company that raised $1 billion led by Alibaba Group;
Humanoid robot company Figure, based in Sunnyvale, California, raised $675 million and partnered with OpenAI; and
Shanghai-based MiniMax, creator of AI companions and avatars, raised $600 million in another round led by Alibaba.
Still, AI was not the largest sector last quarter. The industry that raised the most amount of funding was healthcare and biotech. Companies in that sector raised $15.7 billion, or 24% of all global funding, in Q1.
Seed
Seed companies raised just over $7 billion, down by more than $1 billion year over year.
However, seed funding — which has been the most robust stage through the downturn — is still above 2020 global quarterly amounts. That reflects the fact that during a tightened funding environment, many venture investors looked to invest earlier in the cycle while many founders held off from raising a Series A round as the bar for subsequent investment was raised.
Seed funding counts fell in Q1 2024. (Keep in mind, however, there is typically a pronounced time lag for seed fundings that are added retrospectively to the Crunchbase dataset.)
Early stage grew
Early-stage funding increased in Q1. Funding at this stage totaled around $29.5 billion, up 6% year over year, and was led by large Series B fundings in AI, electric vehicles and green energy, Crunchbase data shows.
Late-stage pullback
Late-stage companies raised $29.5 billion, down 36% year over year, marking the biggest pullback by stage in Q1. In a slower funding market, late-stage funding typically fluctuates quarter over quarter.
IPO markets pop
It is too early to say whether the IPO markets will make a true comeback in 2024. However, two venture-backed companies went public within a day of one another in the latter half of March and both traded up significantly on their first day.
First Cut - State of Private Markets: Q4 2023
Author: Peter Walker
Published date: April 4, 2024
Valuations and round sizes rose at most stages in Q1 2024.
Every quarter, Carta releases information on the startup ecosystem in our State of Private Markets report. It can take a few weeks for rounds to be recorded on our platform, so we produce a full analysis after we get the final numbers.
However, we publish a “first cut” of data as close to the end of the quarter as possible. This initial analysis focuses on round valuations and cash raised across the venture stages.
Here’s a preliminary look at Q4 data for U.S. startups:
Valuations rose—but not for late-stage: Median pre-money valuations ticked upwards for most venture stages, but declined (albeit on low round volume) for Series D and E+.
Round sizes grew: The median amount of cash raised was up for seed, Series A, Series B, and Series C, with particularly sharp increases in the priced seed and Series C stages.
Although the final numbers on total rounds and capital raised are not yet available for Q1, it looks like another quarter of modest growth for venture capital activity is likely.
We’ll publish our full set of quarterly data in the coming weeks. To receive the full report direct to your inbox, sign up for our Data Minute newsletter.
To see the valuations and round size data below split into primary and bridge round figures, you can download the data addendum to the report.
Seed & Series A
Series B & Series C
Series D & Series E+
The Investments Where I’m Going to Lose All My Money
by Jason Lemkin | Blog Posts, Exit Strategy, Fundraising
So when I started writing venture checks in 2013, I didn’t know what I was doing, but I had a strong start:
First was Pipedrive co-leading seed, then acquired for $1.5B cash
Second was Algolia leading U.S. seed, now at $200m+ ARR and an IPO candidate
Third was Greenouse/Parklet, acquired for $800m and now at $200m ARR
Fourth was Salesloft, acquired for $2.5 Billion cash
Fifth was Logikcull, acquired for $300m cash
So it was a good start. Since then, I’ve made some pretty good other investments as well. But also, I made some that … weren’t.
For a while, the 2021 Go Go Days masked everything. Almost everything looked great and had an up-round. But 2024 unmasked a lot of things.
And now, I’m going to lose money on deals. It’s OK at some level. It’s part of the model. But now I can see clearly why in some deals, I’m going to lose all my money.
The top reasons an investment has turned out to be a Zero:
#1. Any misrepresentation about the financials, no matter how small
If the financials are misrepresented even a smidge, I’ve lost all my money or almost all. If revenue was overstated a bit. If contracts were claimed to be closed that weren’t quite closed. Does it really matter? I think it turns out that it does. It just gets worse. I’m going to lose all my money here.
#2. If founders hid anything in the round and/or in diligence. Almost no matter how trivial
Related to but not quite the same as #1. If the founders hide churn, or hide a co-founder is leaving, or really anything that much matters — again, it’s just a smoking gun. It gets worse. They’ll hide even more.
#3. If the founders refuse to get the burn rate under control
OK these ones aren’t always zeros. But they’ve been close to it in my experience. I haven’t done better than 3x here. Note this isn’t just a unicorn issue. It can happen after raising just a few million, too.
#4. If I relied on anyone else being in the round or having already invested, unless they said it was clearly the best deal they are in right now
I haven’t done an investment just because a great investor was already in the deal, but I’ve almost done it. I’ve skipped some steps because of it. Here, I will lose all my money. You have to ignore who else is in the deal.
#5. Not Actually SaaS. Pseudo SaaS but Not Actually SaaS.
I won’t do any of these anymore. The SaaS playbook just doesn’t really work in pseudo-SaaS. Or rather, it seems to work at first, but then it doesn’t really scale.
..More
Quant VC and What it Means for Startup Investing
How Algorithms Shape the Future of VC
APR 04, 2024
The venture capital industry has started to massively professionalize in the past years. We have seen various new models evolving such as boutiques vs asset aggregators, specialists vs generalists, early vs growth vs multi-stage firms, solo vs micro GPs, and a lot more.
One dimension that cuts horizontally through all of these different models is digitization and the use of data & AI. It took 70+ years to start moving from the traditional all-human handcraft setup with manual, inefficient, and oftentimes ineffective workflows to a data-driven model where algorithms and automation augment humans who stay in control of the outcome.
Though less than 1% of VC firms had internal initiatives and experts dedicated to their digital transformation in 2023, we already see the first purist players pushing the limits even further: Quant VC firms. A model that takes the human out of the equation and exclusively relies on data and algorithms, just like Quant Funds did when disrupting the hedge fund industry in the 1990s.
I wrote about the three different models “handcraft VC”, “augmented VC”, and “quant VC” about a year ago here. Since then, I had several controversial discussions about the future of VC and the role of data & AI. While I’m personally a strong believer in an augmented VC approach for early-stage lead investors, I truly enjoy it when others challenge my perspective and have strong arguments to back up their convictions.
Today, I’m incredibly excited to have Guy Conway, the Co-Founder of Quant VC firm Koble.ai who will also join us as a speaker at the Data-driven VC Summit 2024 in early May, share his worldview and why he believes that pure quant strategies will win.
Thank you Guy for your thought-provoking write-up below🌶️🌶️
The AI Backlash Has Already Begun
In recent weeks, negative news headlines have started to proliferate. It seems the media can't make up its mind; journalists hypothesize that AI means death for our species, whilst also claiming that AI is a massive bubble. The schadenfreude from armchair skeptics and full-blown luddites is palpable. It’s almost as if people want us to fail.
What is true, is that the hype around artificial intelligence is normalizing.
According to data compiled by Bloomberg and Apollo chief economist Torsten Sløk, mentions of “AI”, “Machine Learning”, or “Generative AI” on earnings calls decreased from 517 instances in Q4 2023 to just 198 in Q1 2024.
This is healthy, and a net positive for the tech industry, since it reflects the maturation of AI and its wholesale adoption. Just as companies do not claim to be “Internet-driven”, they should not claim to be “AI-driven”, either.
Data Extremism
As the corporate narrative around AI is maturing, so too is the way investors think about integrating data and AI into their operations. The application of Data Science to the dark art of Venture Capital – and its impact on the investment process – is still nascent, and will take many years before its true impact can be measured and understood.
VCs have made decent progress in adopting tools to streamline operations and bring more rigor to the investment process, which remains stubbornly human. But how much further can Data-driven VCs go before hitting a ceiling in terms of how much internal spending can be allocated to refreshing the data stack?
And even if spending on data was unlimited, there is still the immovable (and seemingly ubiquitous) belief that data is the input; only humans can run the Investment Committee. How strange that we let AI discover new drugs for us, and then diagnose us with the illnesses with which those drugs should treat us, but the widely held industry belief remains that AI can’t tell us if a startup investment is the right one!
A growing subset of investors is calling for a more hardcore approach – one that excludes humans from the investment process entirely. But this camp is very much in the minority.
Full automation of the startup selection process requires technology that’s orders of magnitude more complex and capable than that which is currently accessible to mainstream VCs. But purist (some might say, extremist) “Quantitative VCs” are quietly building in the background.
Going Beyond the Consensus
AI is perfectly suited to helping humans manage informational overabundance, and deconstruct the Power Law to demystify the “miracle” of Venture Capital. Use cases are plentiful and well documented:
Deal flow filtering – analyse large datasets of startups, identifying patterns, trends, and KPIs and allowing fund managers to filter and prioritise investment opportunities.
Due diligence – conduct due diligence on startups by analysing vast amounts of unstructured data, such as news articles, social media posts, industry reports, and financial statements.
Predictive analytics – leverage data to develop predictive models that estimate the success or failure of startups.
Portfolio Management – manage portfolios more effectively by continuously monitoring and optimising the performance of existing investments.
Domain expertise – provide contextualised insights at the company and industry level, simulating and scaling the expertise of practitioners.
Taken as a whole, these are significant upgrades to the status quo. But Quant VCs argue they can go further, disrupting old-school investment methodologies and reliably hitting the upper echelons of the asset class.
What we've seen with Data-driven VC is about sourcing and discovery and picking off the low-hanging fruit of automating internal processes.
Quant VC goes much further – it’s about investment selection and portfolio construction too. In the long term, there’s a drive toward automation of the end-to-end capital deployment process.
The mean return in early-stage VC over 20 years is 21.3% IRR, yet median returns are at 5% IRR. No asset class (other than Crypto) can even get close to that. Adoption of Quant VC means we should consistently be able to hit the juicy returns the asset class offers. And that comes down to being better at picking the winners and the science of portfolio construction. Most VCs fail to do this.
History Repeating
Years ago, hedge funds disrupted public markets with data science. In the public markets, algorithmic trading decisions now represent up to 75%+ of total trading and AUM of $1+ trillion.
The history of the hedge fund industry can teach us much about the future of Venture Capital. Jim Simons – the billionaire founder of quantitative hedge fund Renaissance Technologies – is perhaps the greatest money-maker in modern financial history, with a track record for value accretion that surpasses Warren Buffett, George Soros and Ray Dalio.
Video of the Week
AI of the Week
Meet the YC Winter 2024 Batch
by Garry Tan 4/3/2024
Today we’re kicking off Y Combinator’s 38th Demo Day, celebrating the incredible batch that is W24. This batch marks the completion of my first year as President of YC — and what an amazing year it’s been.
During the last batch we moved Y Combinator into a beautiful office in San Francisco’s Dogpatch neighborhood. This batch we took over a massive building next door, too — more than tripling the space available to us.
But this expansion has meant so much more than just additional square footage. What we call “the office” has very quickly become more of a campus, and the energy of this campus is unlike anything else in startup land. Hundreds of YC founders are coming through these doors each and every day for office hours, meetups, and just to be among their fellow builders. As Jared Friedman put it recently: YC’s new home is the most concentrated focal point the early stage startup ecosystem has ever seen.
Good energy begets good energy, and the energy from our office is already spilling out in great ways to the neighborhood around it. Many of the founders who came to SF for the batch have decided to make SF home — for themselves, and for their companies. We are turning San Francisco’s doom loop into a boom loop.
A new office also means new traditions. Just one example of a tradition that began this batch: Bookface Launch Live.
Imagine a room where the best builders in the world are presenting the fruits of their labor, often weeks or months before they show it anywhere else. A room full of builders showing each other what is possible, peering into the future together, and collaborating to get there even faster. That’s Bookface Launch Live.
As YC’s office footprint has grown, so has YC as an organization. YC’s roster of Group Partners is stronger than it has ever been, and we expect that roster to only grow. These are the people hand-picking the companies YC invests in, working with them throughout the batch, and supporting these founders for the life of their company.
As he shared a few weeks ago, Michael Seibel is returning to his original role as a YC Group Partner — a move that lets him focus more of his time on the job he likes most: mentoring founders. The list of what Michael has accomplished for YC is miles long; if something is an important part of YC, he likely played a key part in it. We all thank you, Michael, and we’re honored that we get to continue working with you for the next many years.
This batch we also welcomed Pete Koomen (founder of Optimizely, an absolute juggernaut of a startup from W10) as a Group Partner, as well as Tyler Bosmeny (Clever, S12) and Nate Smith (Lever, S12) as Visiting Group Partners. Expect more announcements here soon.
260 companies joined YC for W24, as selected from over 27,000 applications. With an acceptance rate under 1%, this was one of the most selective cohorts in YC history.
The 18 most interesting startups from YC’s Demo Day show we’re in an AI bubble
Dominic-Madori Davis, Tim De Chant, Rebecca Szkutak, Marina Temkin, Kyle Wiggers, Alex Wilhelm/ 12:44 PM PDT•April 3, 2024
Image Credits: Bryce Durbin/TechCrunch
Springtime means rain, the return of flowers and, of course, Y Combinator’s first demo day of the year. During the well-known accelerator’s first of two pitch days from the Winter 2024 cohort, a covey of TechCrunch staff tuned in, took notes, traded jokes and slowly whittled away at the dozens of presenting companies to come up with a list of early favorites.
AI was, not shockingly, the biggest theme, with 86 out of 247 companies calling themselves an AI startup, but we’re reaching bubble territory given that 187 mention AI in their pitches.
From AI-generated music and grant applications to neat new fintech applications and even some health tech work, there was something for everyone. We’re back at it Thursday for the second day of pitches. Until then, if you didn’t get to watch live, here’s a rundown of some of the best from day one.
TechCrunch’s staff favorites
Aidy
What it does: Uses AI to help companies find and apply for grants
Why it’s a fave: Landing grants isn’t easy. Max Williamson, Peter Crocker and Greg Miller know this well: They’ve worked between them at The Rockefeller Foundation and the U.S. Department of Housing and Urban Development, where grants are common currency. Finding and applying for grants involves sifting through mounds of paperwork and submitting countless forms — an expensive and time-consuming process. So why not have AI help with it? That’s the idea behind their startup Aidy, which is focused exclusively on Rural Energy for America Program grants for now. After asking a few questions, Aidy evaluates an organization’s competitiveness for grants by navigating eligibility requirements and scoring criteria, then takes a first pass at filling out any relevant forms. Aidy is clearly in the proof-of-concept stage, judging by the state of its tooling. But the concept’s an interesting one — assuming the platform’s AI doesn’t make too many mistakes.
Who picked it: Kyle
Givefront
What it does: Serves as a banking platform for nonprofits
Why it’s a fave: If you’re in the nonprofit space, compliance and regulatory requirements force you to do finances a little differently. That’s where Givefront comes in. Co-founded by Ethan Sayre and Matt Tengtrakool, who previously launched a startup to help loan-takers based in Nigeria, Givefront offers banking, spend management and financial governance services for nonprofits. Specifically, Givefront provides accounts to nonprofits to store money and integrate donations, payments and reimbursements, as well as features for automatic reporting and annual regulatory filings. Givefront certainly isn’t the only nonprofit banking option out there. But it appears to be one of the first built from the ground up for this purpose — which certainly has its own appeal.
Who picked it: Kyle
YCombinator's AI boom is still going strong (W24)
Combing through all 158 YC AI startups (65% of the batch).
APR 03, 2024
With YC's latest Demo Day (W24), the AI companies are continuing to grow. Six months ago, there were around 139 companies working with AI or ML - that number has climbed to 158, a clear majority of 65% (there are 243 total companies in the batch).
Let's dive into what's new, what's stayed the same, and what we can learn about the state of AI startups.
The biggest domains stayed big
Perhaps unsurprisingly, the most popular categories remained unchanged from the last batch. Last time, the top 4 domains were AI Ops, Developer Tools, Healthcare + Biotech, and Finance + Payments. This time, the top 5 were:
Developer Tools: Apps, plugins, and SDKs making it easier to write code. Tools for testing automation, website optimization, codebase search, improved Jupyter notebooks, and AI-powered DevOps were all present. There was also a strong contingent of code-generation tools, from coding Copilots to no-code app builders.
AI Ops: Tooling and platforms to help companies deploy working AI models. That includes hosting, testing, data management, security, RAG infrastructure, hallucination mitigation, and more. We'll discuss how the AI Ops sector has continued to mature below.
Healthcare + Biotech: While I've once again lumped these two categories together, there's a pretty big split in the types of AI businesses being built. Healthcare companies are building automation tools for the entire healthcare lifecycle: patient booking, reception, diagnosis, treatment, and follow-up. Whereas biotech companies are creating foundation models to enable faster R&D.
Sales + Marketing: Early generative AI companies were focused on the sales and marketing benefits of GPT-3: write reasonable sounding copy instantly. Now, we're seeing more niche use cases for revenue-generating AI: AI-powered CRMs for investors, customer conversation analysis, and AI personal network analysis were among some sales-oriented companies.
Finance: Likewise, on the finance side, companies covered compliance, due diligence, deliverable automation, and more. Perhaps one of my favorite descriptions was "a universal API for tax documents."
The long tail is getting longer
Even though the top categories were quite similar, one new aspect was a wider distribution of industries. Compared with the last batch, there were roughly 35 categories of companies versus 28 (examples of new categories include HR, Recruiting, and Aerospace). That makes sense to me. I've been saying for a while now that “AI isn't a silver bullet” and that you need domain-expertise to capture users and solve new problems.
But it's also clear that with AI eating the world, we're also creating new problems. It was interesting to see companies in the batch focused on AI Safety - one company is working on fraud and deepfake detection, while another is building foundation models that are easy to align. I suspect we will continue seeing more companies dealing with the second-order effects of our new AI capabilities.
..More
Bubble Trouble
EDWARD ZITRON APR 4, 2024
As I previously warned, artificial intelligence companies are running out of data. A Wall Street Journal piece from this week has sounded the alarm that some believe AI models will run out of "high-quality text-based data" within the next two years in what an AI researcher called "a frontier research problem."
Modern AI models are trained by feeding them "publicly-available" text from the internet, scraped from billions of websites (everything from Wikipedia to Tumblr, to Reddit), which the model then uses to discern patterns and, in turn, answer questions based on the probability of an answer being correct.
Theoretically, the more training data that these models receive, the more accurate their responses will be, or at least that's what the major AI companies would have you believe. Yet AI researcher Pablo Villalobos told the Journal that he believes that GPT-5 (OpenAI's next model) will require at least five times the training data of GPT-4. In layman's terms, these machines require tons of information to discern what the "right" answer to a prompt is, and "rightness" can only be derived from seeing lots of examples of what "right" looks like.
While the internet may feel limitless, Villalobos told the Journal that only a tenth of the most-commonly-used web dataset (the Common Crawl) is actually "high quality" enough data for models. Yet I can find no clear definition of what "high-quality" even means, or proof that any of these companies are being picky with what they train their data on, only that they have an insatiable hunger for more data, relying instead on thousands of underpaid contractors (with some abroad making less than $2 an hour, a growing human rights crisis in and of itself) to teach their models how to say and do the right thing when asked.
In essence, the AI boom requires more high-quality data than currently exists to progress past the point we're currently at, which is one where the outputs of generative AI are deeply unreliable. The amount of data it needs is several multitudes more than currently exists at a time when algorithms are happily-promoting and encouraging AI-generated slop, and thousands of human journalists have lost their jobs, with others being forced to create generic search-engine-optimized slop. One (very) funny idea posed by the Journal's piece is that AI companies are creating their own "synthetic" data to train their models, a "computer-science version of inbreeding" that Jathan Sadowski calls Habsburg AI.
This is, of course, a terrible idea. A research paper from last year found that feeding model-generated data to models creates "model collapse" — a "degenerative learning process where models start forgetting improbable events over time as the model becomes poisoned with its own projection of reality."
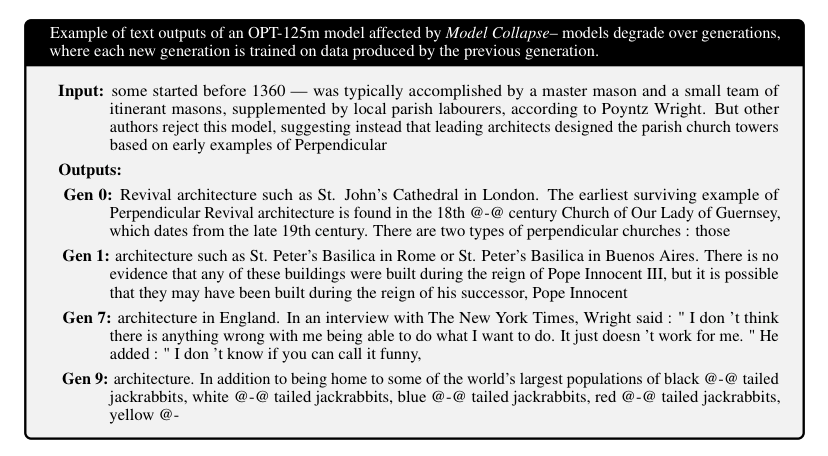
AI models, when fed content from other AI models (or their own), begin to forget (for lack of a better word) the meaning and information derived from the original content, which two of the paper's authors describe as "absorbing the misunderstanding of the models that generated the data before them."
In far simpler terms, these models infer rules from the content they're fed, identifying meaning and conventions as a result based on the commonalities of how humans structure things like code or language. As generative AI does not "know" anything, when fed reams of content generated by other models, they begin learning rules based on content generated by a machine guessing at what it should be writing rather than communicating meaning, making it somewhere between useless and actively harmful as training data.
Sidebar: I spoke with Zakhar Shumaylov, PhD Student at the Department of Mathematics at the University of Cambridge, and one of the authors ofthe original model collapse paper. He believes that model collapse is already happening, and training with this generated data compounds existent biases in a model and, unfortunately, errors. One interesting note he made was that human-made training data, by the nature of it being written by a human, includes errors, and models need to be robust to such errors. So what do we do if models are trained with content created without them? Do we introduce errors ourselves? How many errors are there, and how do we introduce them all?
It's tough to express how deeply dangerous this is for AI. Models like ChatGPT and Claude are deeply dependent on training data to improve their outputs, and their very existence is actively impeding the creation of the very thing they need to survive. While publishers like Axel Springer have cut deals to license their companies' data to ChatGPT for training purposes, this money isn't flowing to the writers that create the content that OpenAI and Anthropic need to grow their models much further. It's also worth considering that these AI companies may already have already trained on this data. The Times sued OpenAI late last year for training itself on "millions" of articles, and I'd bet money that ChatGPT was trained on multiple Axel Springer publications along with anything else it could find publicly-available on the web.
Big Tech companies form new consortium to allay fears of AI job takeovers
Kyle Wiggers @kyle_l_wiggers / 4:30 AM PDT•April 4, 2024
Image Credits: Anastasia Usenko / Getty Images
AI might not be coming for all jobs, but it might be coming for some.
UPS’s largest layoff in its 116-year history was the result of, in part, new technologies, including AI, CEO Carol Tomé said during an earnings call in February. Meanwhile, IBM plans to pause hiring for roles it thinks could soon be automated by AI, CEO Arvind Krishna told Bloomberg last year.
Workers aren’t optimistic about the future. In a recent survey from McKinsey, 25% of business professionals said that they expect their employer to lay off staff as a result of AI adoption. And, well, their pessimism isn’t misplaced. According to one estimate, around 4,000 workers have lost their jobs to AI since May. And in a poll from Beautiful.ai, which makes AI-powered presentation software, nearly half of managers said that they’re hoping to replace workers with AI.
But a cohort of Big Tech vendors and consultancies — called the AI-Enabled ICT Workforce Consortium (ITC) — aims to push back against the notion that AI will lead to job losses, citing the need for re-skilling and upskilling within the information and communication technology (ICT) industry specifically.
The ITC is being led by Cisco with support from Google, Microsoft, IBM (conspicuously), Intel, SAP and Accenture. The ITC’s mandate is to explore AI’s impact on jobs while enabling people to find AI-related training programs and connecting businesses to “skilled and job-ready” workers, a spokesperson told TechCrunch in a briefing.
“The ITC’s unique approach will research and evaluate the impact of AI on specific job roles, including skills and tasks, and recommend training for an AI-enabled ICT workforce,” the spokesperson said. “Consortium members and advisers share a common perspective that a greater sense of urgency is required to understand the impact of AI on key job roles within the ICT Industry.”
In the first phase of its work, the ITC will evaluate the impact of AI on 56 ICT job roles and provide training recommendations for the roles affected. These 56 roles, which the ITC hasn’t disclosed yet, were selected for their “strategic significance” in the broader ICT ecosystem and AI’s impact on the tasks required to perform the roles, the spokesperson said, as well as roles that offer “promising entry points” for low-level workers.
News Of the Week
Apple Vision Pro’s Persona feature gets collaborative
Brian Heater @bheater / 11:33 AM PDT•April 2, 2024
Image Credits: Apple
Much like the headset for which they were designed, Apple’s Personas are very much a work in progress. The original version of the beta avatars were — is “nightmarish” too strong a word? A subsequent update has made them more palatable and truer to life, and Apple says it’s continuing to work on the 3D captures.
The company on Tuesday debuted “spatial” Personas for Vision Pro headsets running visionOS 1.1 or later. Whereas the feature was previously limited to chat platforms like FaceTime and Zoom, the new version is designed to bring an added sense of collaboration to the headset.
The spatial aspect still starts with FaceTime, but Apple’s proprietary videoconferencing platform now serves as a gateway to other apps when combined with SharePlay. From there, users can select the spatial persona option, which utilizes the Vision Pro’s on-board sensors to place the Persona in the room with them.
Spatial audio, meanwhile, further places them at a specific point in space relative to the Vision Pro user.
In the video example shared by Apple, two Personas flank a window showcasing Freeform. Taken together, this approximates the sense of people collaborating on a project across an office conference table. In this case, however, that conference table is the user’s desk at home.
Is the effect neat? Yes. Is it creepy? Also, yes. Vision Pro users will continue doing business in the uncanny valley for the foreseeable future. That just comes with the territory of being an early adopter.
Jon Stewart Plunges the Knife into Apple
Hypothetical: let's say you're a multi-trillion dollar company under various business practice investigations from all sides, all around the world. Would it be a good thing to see your company name in the press for allegedly blocking one of the biggest names in entertainment, on your payroll, from interviewing the most well-known figurehead of the movement coming after you? Such is the position Apple amazingly finds itself in today with the report that the company blocked Jon Stewart from interviewing FTC Chair Lina Khan.
Yes, this is probably the worst possible headline for Apple, at the worst possible time.
And don't think Jon Stewart isn't aware of that. I'm sure all sides will deny it, but payback is nevertheless a bitch. And this sure seems to be Stewart digging a knife into Apple right after the announcement of the DoJ's lawsuit against the company for illegal monopolistic behavior. I mean, he had Lina Khan on as his Daily Show guest this weekand said to her (16:32 in):
"I gotta tell you. I wanted to have you on a podcast. And Apple... asked us not to do it. To have you. They literally said 'please don't talk to her.' Having nothing to do with what you do for a living, I think they just... (laughs) I didn't think they cared for you, is what happened. They wouldn't let us do even that dumb thing we just did in the first act on AI. Like, what is that sensitivity? Why are they so afraid to even have these conversations out in the public sphere?"
Khan's response, was the sort of typical generic thing about the concentration of power in the market being a bad thing. But Stewart's words are the real story here. And the fact that he was saying them to her. Yes, she's more focused with the FTC, at the moment, on Amazon (which the interview is mainly about) with the DoJ taking on Apple. But Stewart is basically saying, "you gotta get these guys."
As Axios notes:
The complaint specifically calls out Apple's dominance as potentially harmful to speech:
"Apple's conduct extends beyond just monopoly profits and even affects the flow of speech. For example, Apple is rapidly expanding its role as a TV and movie producer and had exercised that role to control content," it notes.
..More
Startup of the Week
Rubrik’s IPO filing hints at thawing public markets for tech companies
Alex Wilhelm, Rebecca Szkutak, Marina Temkin/ 1:27 PM PDT•April 2, 2024
Image Credits: krblokhin / Getty Images
Rubrik, a data cybersecurity company that raised more than a half-billion dollars while private, filed to go public after the bell on Monday. Following quickly on the heels of debuts from Reddit and Astera Labs, the choice by Rubrik to pursue a public offering now could indicate that the IPO market is warming for tech companies.
As a private-market company, Rubrik last raised a lettered round in 2019 when it closed $261 million at a $3.3 billion post-money valuation, according to Crunchbase data. The company could have luck pricing its IPO shares significantly higher than its last primary round. Buyers on the secondary market have bid for shares valuing the company at $6.6 billion in recent months. Secondary data platform Caplight estimates the company’s valuation hovers around $6.3 billion.
Rubrik sells its cloud-based data protection platform to enterprises. As of January, the company had over 1,700 customers with an annual contract value of $100,000 and nearly 100 customers who paid Rubrik over $1 million a year, according to its IPO filing.
Inside Rubrik’s growth
Rubrik initially presents as a moderately growing software business with net losses that stretched to $354 million in its most recent fiscal year.
From its fiscal 2023 to its fiscal 2024, which concluded at the end of January this year, the company’s revenue grew from $599.8 million to $627.9 million, or just under 5%. However, subscription revenue grew 40% over the same period, rising from $385.3 million to $537.9 million.
The growth in its subscription revenue, and not its legacy revenues, is the engine that could propel Rubrik to a successful IPO. The company began life as a software company that sold its product on a perpetual license basis. However, after several years, it began to shift toward a subscription model in its fiscal 2019. It expanded its subscription (SaaS) offerings over time and told investors in its IPO filing that it anticipates that its non-recurring revenues will “continue to decrease,” as it doesn’t generally offer perpetual licenses today.
Rubrik’s transformation to recurring revenues is nearing its completion, with the company reporting that in its most recent quarter — the period ending January 31, 2024 — subscription-related top line comprised 91% of its total revenue. That was up from 73% in the year-ago quarter.
..More
When is a Bubble Not a Bubble?